Breadcrumb
AI ‘hallucination’ research led by Yarin Gal and team appears in Nature
An article by Professor Yarin Gal, Tutor in Computer Science at Christ Church, and members of his Oxford Applied and Theoretical Machine Learning Group (OATML) has been published today in Nature. The research of Professor Gal, Dr Sebastian Farquhar, Jannik Kossen and Lorenz Kuhn proposes a means of predicting when large language model (LLM) systems such as ChatGPT and Gemini are likely to ‘hallucinate’ – that is, invent intuitively plausible but wholly imaginary facts.
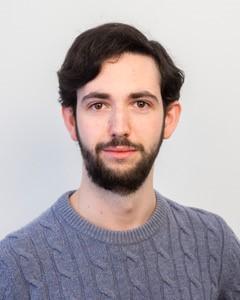
ChatGPT, Gemini and other LLMs have widely been seen as a triumph of AI research, unlocking huge investment. Yet for all of their impressive reasoning and question-answering capabilities, the systems are regularly found to ‘hallucinate’ credible but false or unsubstantiated outputs. This problem of hallucination poses risks in contexts in which the truth really matters – for instance, when conducting legal research or medical diagnosis.
Today’s Nature article sets out a way in which we can spot when LLMs are likely to make up its answers. Just as a bad liar will tell inconsistent stories, the authors hypothesise that in many instances a hallucinating LLM will generate different answers each time you ask – known as ‘confabulating’.
However, as one of the authors Dr Sebastian Farquhar explains, gauging this inconsistency in the case of LLMs is challenging: ‘The creativity of generative models is a double-edged sword. LLMs can phrase the same thing in many different ways. Old approaches couldn’t tell the difference between a model being uncertain about what to say versus being uncertain about how to say it.’
In situations where reliability matters, computing semantic uncertainty is a small price to pay.
In situations where reliability matters, computing semantic uncertainty is a small price to pay.
This is the advance made by Professor Gal and his co-authors: the team have developed a way of identifying when LLMs are uncertain about the particular meaning of an answer, not just the phrasing. The solution is to translate probabilities associated with the words (produced by the LLMs) into probabilities associated with meanings. ‘The trick is to estimate probabilities in meaning-space, or “semantic probabilities”,’ says Jannik Kossen, one of the article’s contributors. ‘We show how to use LLMs themselves to do this conversion.’
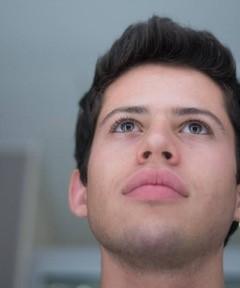
This method is more computationally costly than using LLMs in the usual way, but as Professor Gal reminds us, ‘In situations where reliability matters, computing semantic uncertainty is a small price to pay.’ This is the latest research to come out of Oxford Applied and Theoretical Machine Learning Group, led by Professor Gal, which is pushing at the frontiers of robust and reliable generative models. The group’s research complements Professor Gal’s work in the UK Government’s AI Safety Institute, formerly the Frontier AI Taskforce, of which he was made the first Research Director last year. In both groups, Professor Gal seeks to address what he sees as ‘an urgent need for research that measures and mitigates emerging risks from generative AI.’
While the Oxford team’s findings will help us to eliminate hallucinations associated with confabulation, there is plenty of work to be done to address the many other types of error that LLMs can make. As Dr Farquhar explains, ‘Semantic uncertainty helps with specific reliability problems, but this is only part of the story. If an LLM makes consistent mistakes, this won't catch that.
‘The most dangerous failures of AI come when a system does something bad but is confident and systematic. There is still a lot of work to do.’
Yarin Gal is Tutor in Computer Science at Christ Church, group leader for the Oxford Applied and Theoretical Machine Learning Group (OATML) in the Department of Computer Science, and Research Director of the UK Government’s AI Safety Institute. Learn more about his academic background and research via his Christ Church profile.
Sebastian Farquhar is a former Tutor in Computer Science at Christ Church and is now an associate member Senior Research Fellow at the Oxford Applied and Theoretical Machine Learning Group (OATML) and a Senior Research Scientist at Google DeepMind. Learn more about his work from his OATML profile.
Other Christ Church news
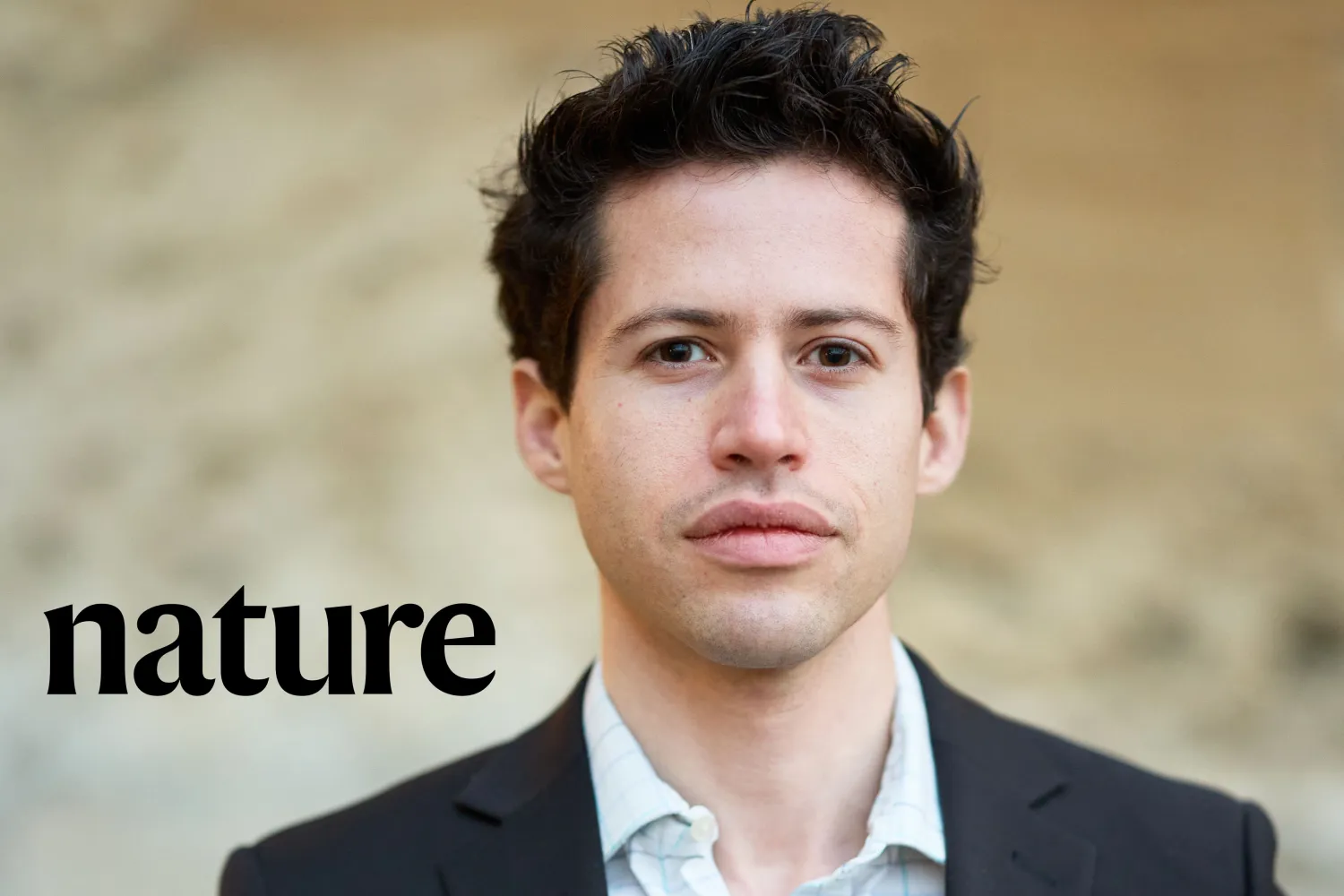