Breadcrumb
Could machine learning models cause their own collapse?
AI systems have shown remarkable advances in recent years, but an article published today in Nature highlights a potential stumbling block on the horizon for them. The paper’s authors, who include Christ Church’s Dr Ilia Shumailov and Professor Yarin Gal, set out how the ‘indiscriminate’ training of Large Language Models such as ChatGPT and Gemini on model-generated data ‘causes irreversible defects in the resulting models’ – giving rise to a phenomenon the researchers term ‘model collapse’.
In the last few years the prevailing story told about large language models (LLMs) such as OpenAI’s ChatGPT and Google’s Gemini has been one of breathtaking advancements and the proliferation of use cases – from drafting emails and reports, to generating images and poetry. These leaps in performance are generally attributed to the development of more efficient hardware and the training of models on a growing pool of high-quality data. The largest models today have even been trained on the entire human-produced Internet.
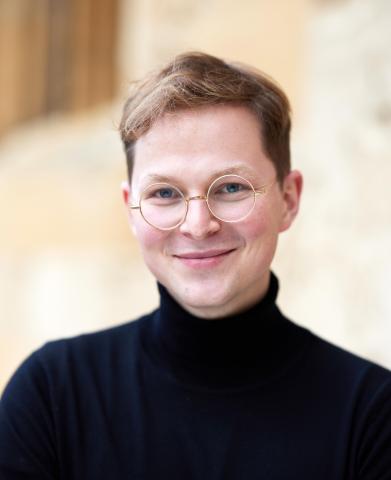
Yet while hardware is forever improving, the same cannot be said of data. The explosion in the use of LLMs has precipitated a shift in the online ecosystem, with a growing share of data being produced by these AI systems, free from human oversight. What is the effect of training LLMs on such artificial AI-generated data? This is the question that Dr Ilia Shumailov, lead author of today’s paper, sought to answer in research completed as a Junior Research Fellow (JRF) in Computer Science at Christ Church, and as a member of Professor Yarin Gal’s Oxford Applied and Theoretical Machine Learning Group (OATML).
Model collapse refers to AI spiralling into the abyss, feeding on its own mistakes and becoming increasingly clueless and repetitive.
Model collapse refers to AI spiralling into the abyss, feeding on its own mistakes and becoming increasingly clueless and repetitive.
Today’s article sets out the sobering findings of Dr Shumailov’s research, completed in collaboration with Zakhar Shumaylov and Professor Ross Anderson of the University of Cambridge, Dr Yiren Zhao of Imperial College London, Professor Nicolas Papernot of the University of Toronto, and Christ Church’s Professor Gal. The researchers find that training LLMs on LLM-generated data can cause the ‘collapse’ of such models – that is, when the machine learning models ingest recursively generated data the models severely degrade in quality.
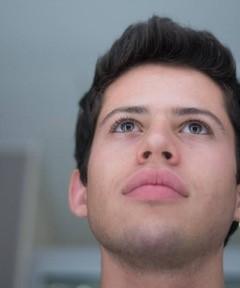
The source of the problem is the minor errors generated by all machine learning models. Such errors are reproduced by subsequently trained models, which in turn add slight errors of their own. In this way, we see a compounding of errors that ultimately breaks LLMs. As Dr Shumailov explains, what we see is ‘model collapse’, where this ‘refers to AI spiralling into the abyss, feeding on its own mistakes and becoming increasingly clueless and repetitive.’
The moral of the story is that future LLM development requires access to original, human-generated data in order to avert model collapse. There must be human monitoring and curation of the data that is being fed into machine learning models, and the source of data must be identified. As it becomes increasingly difficult to tell human-produced data from its AI-generated counterpart, the task of staving off model collapse promises to become ever more challenging.
The paper from Dr Shumailov, Professor Gal and their co-authors can now be read online.
Yarin Gal is Tutor in Computer Science at Christ Church, group leader for the Oxford Applied and Theoretical Machine Learning Group (OATML) in the Department of Computer Science, and Research Director of the UK Government’s AI Safety Institute. Learn more about his academic background and research via his Christ Church profile.
Ilia Shumailov recently completed his tenure as a Junior Research Fellow in Computer Science at Christ Church and is an Associate Member of the Oxford Applied and Theoretical Machine Learning Group (OATML). A list of his publications can be found on his Google Scholar profile.
Other Christ Church news
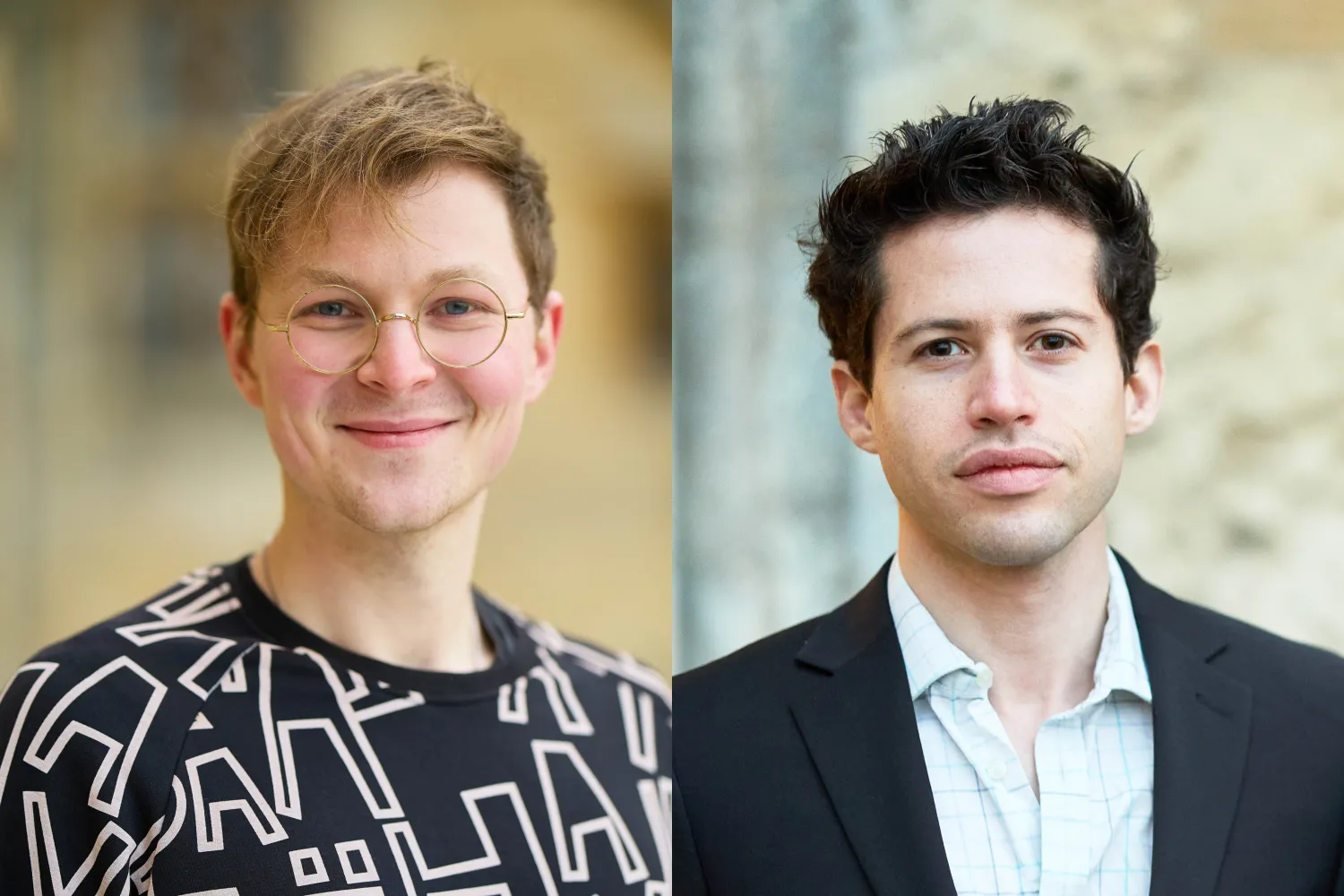